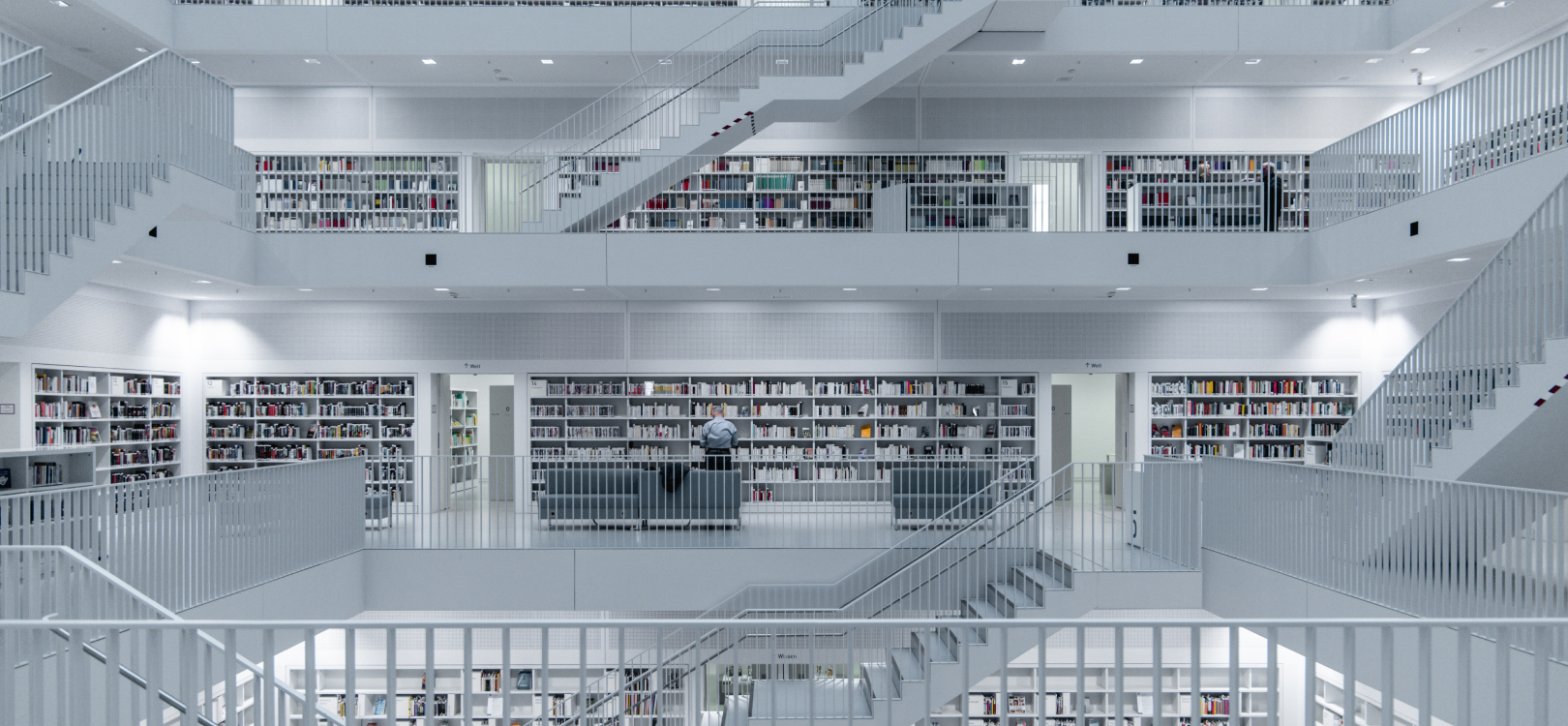
Advantages of Big Data:
-
real-time data analysis
the ability to make more informed business decisions
increasing the competitive advantage
organization, storage, and management of data sets
What we offer:
Big Data services for any business
complete solutions exclusively for your needs
we turn unstructured data into useful knowledge
Applied technologies:
Machine Learning
web scraping
data encryption
AI
We provide tailor-made solutions
for our customers
To learn more about our services and request price,
please, contact us and tell about your purpose.
Popular questions about Bigdata platform
A big data platform is a type of IT solution that combines the features and capabilities
of multiple big data applications and utilities into a single solution.
It is an enterprise-class IT platform that enables an organization to design, deploy,
operate, and manage a big data infrastructure/environment.
A big data platform typically consists of big data storage, servers, databases, big data
management, business intelligence, and other big data management utilities. It also
supports custom developments, queries, and integration with other systems. The main
benefit of a big data platform is to reduce the complexity of multiple vendors/solutions
into one cohesive solution. The big data platform is also delivered via the cloud, where
the provider provides end-to-end big data solutions and services.
It can be conditionally divided into the following stages:
Data collection. It can be open and open sources. The former include: data from public
services, publicly available commercial information, social networks, Internet services.
Ko husband is an analyst, data on online transactions). For the transmission of standard
application interfaces and protocols.
Data integration. Special systems convert them to a format suitable for storage, or
limit tracking to discuss triggers.
Processing and analysis. Operations in real time except when the information is logged
to the video functions for processing. Popular analysis techniques: training in
associative procedures, classification, cluster and regression analysis, mixing and
combining data, machine learning, pattern recognition, and others.
An important element of working with big data is search, which allows you to get data
about fibers. In a simple case, this works just like Google. Data is obtained for
external and external parties for a fee or free of charge - it all depends on the
conditions of ownership of them. Big data is in demand among enterprises and application
services, trade and telecommunications companies. For business users, information is
collected in a visualized form that is easy to understand. If the format is text, then
these will be concise lists and excerpts; if the format is graphic - graphics, graphics
and animation.
Structured data. It is the simplest data type in terms of organization and retrieval.
This includes, for example, financial data, equipment logs, and demographic data. An
Excel spreadsheet with predefined columns and rows is a convenient way to present
structured data. Its components can be easily categorized, allowing developers and
database administrators to define simple search and analysis algorithms. But even if
structured data is huge, it is not necessarily big data because structured data itself
is relatively easy to manage and therefore does not meet the defining criteria of big
data. Traditionally, a programming language called the Structured Query Language (SQL)
has been used to manipulate structured data stored in a database. Developed by IBM in
the 1970s, the SQL language is intended to enable developers to create relational
databases (in the spreadsheet style) that were beginning to gain popularity during this
period.
Unstructured data. This category of data may include items such as social media posts,
audio files, images, and customer comments with open-ended questions. This kind of data
is not easy to organize into standard relational databases with columns and rows.
Traditionally, companies that needed to search, manage, or analyze large amounts of
unstructured data had to use time-consuming manual processes. The potential value of
analyzing and understanding such data was never questioned, but the cost of analysis was
often prohibitive, making it impractical. Due to the high processing time, the results
were often out of date before they were received. Instead of spreadsheets or relational
databases, unstructured data is typically stored in data lakes, data warehouses, and
NoSQL databases.
semi-structured data. As the name implies, semi-structured data is a hybrid of
structured and unstructured data. Email messages are a prime example: they can include
unstructured data in the body of the message, as well as organizational parameters such
as sender, recipient, subject, and date. Devices using geolocation, timestamps, or
semantic tags can also provide structured data along with unstructured content. For
example, an unidentified image from a smartphone can provide information about its
category (selfie), as well as the time and place it was taken. A modern AI-powered
database can not only instantly identify different types of data, but also generate
algorithms in real time to effectively manage and analyze disparate data sets.
"Volume". By 2020, the total volume of information created in the digital environment has
reached 44 zettabytes. The World Economic Forum predicts that by 2025, the volume of
daily Internet data traffic worldwide will reach 463 exabytes. In terms of a visual
assessment of such a huge amount of information, it should be noted that it would take
more than 212 million DVDs to record it. The information that constitutes the volume of
"big data" comes from the millions of electronic networked devices and applications in
use. For example, in 2019, the average daily number of created messages on Twitter was
500 million, 294 billion emails, 65 billion messages and 2 billion voice recordings in
the WhatsApp messenger, 5 billion search queries, etc. It is important to keep in mind
that at the stage of information accumulation, Big Data does not select "unnecessary"
data: any transactions, user actions on the network (including just browsing Internet
pages, without taking active actions or clicking on links).
Conventional storage and analysis tools are not capable of handling this amount of data.
"Velocity". The above data volumes are processed in real time, as opposed to traditional
batch data processing. This means that they accumulate instantly, while the duration of
the data stream itself does not matter. Thus, Big Data not only captures data flows, but
also records and processes them in such a way that there are no losses. An example of
streaming data processing is the YouTube service, which analyzes user data based not
only on fully watched videos and broadcasts, but also on materials that users skipped
and perceived as unnecessary. For the purposes of channel creators, YouTube additionally
offers services for collecting data on viewers' interests, geographic features, content
preferences, suggestions for the target audience9.
"Variety" Big Data is formed from various sources and in the form of a wide variety of
data formats (video data, photographs, sound recordings, text messages, transaction
files, comments, link usage and page view capture, etc.). The largest amount of "big
data" is formed from information in social networks and social media services and
represents either partially structured or unstructured information.
Sometimes Data Science platforms are perceived as analogues of Business Intelligence (BI)
systems, as they also contain tools for visualizing the results of data analysis. It is
important to understand the differences between them in order to choose the scope.
Traditionally, BI solutions are used for static reporting on the current or past state
of a business. They answer questions such as:
What is the dynamics of sales in the last quarter?
What caused the increase or decrease in sales?
what type of product produced the most in a month?
This is the so-called descriptive or descriptive analysis. In addition, BI systems work
with structured data extracted from data warehouses and present the result of the
analysis in the form of interactive dashboards - dashboards or reports.
Big data analysis platforms are already a tool for predictive and dynamic analysis. They
allow you to make forecasts for the development of any business area and make more
accurate decisions based on them. Typical questions: what is the optimal business
development scenario? What happens if current trends continue? What happens if a new
managerial decision is made?
Platforms can use both structured and unstructured data from multiple sources, and are
adept at handling big data. Since predictive analysis is aimed at predicting some
parameter or event, it focuses on a specific task, unlike business intelligence.
Descriptive analysis, on the other hand, should allow users the flexibility to create a
report in the context that they need.
Modern BI systems have a wide range of visualization tools: from line charts and pie
charts to heat maps and sledge charts. Therefore, although BI systems and data science
platforms are designed for different tasks, they can complement each other. For example,
an existing BI system can conveniently present the results of the analysis of data that
comes from the platform.
Usually in a company, each team has a tool for streamlining processes and collaborating
on tasks. Data processing platforms are as necessary a tool for data scientists as a
source code control system for developers, CRM for sales department and helpdesk for
technical support.
For example, in small companies, excel files or cloud kanban services are used instead
of CRM. Different managers may use different tools. At a certain stage, problems arise:
information is not stored in one place, managers do not have a single access to it,
files increase in volume, it takes a long time to search for information in them, and it
is difficult to scale such a system.
Similar difficulties arise in data science projects. Here are signs that it's time for
you to start using a big data analytics platform:
Reduced productivity. If you notice that there are too many tools, you spend a lot of
time on routine tasks, and the time for data processing and maintenance of different
tools is only growing - most likely, it's time for you to switch to the DS platform.
Scaling difficulties. When you need to replicate the developed application to other
lines of business or one of the stages of data processing required more computing
resources, then there is a need for scaling. If you need to scale, but you don't have a
very good idea of how to do it, then a platform is a must.
Transparency. If there are problems with communication in a team of data scientists,
then this indicates a lack of centralized knowledge that can be easily shared among
themselves. Modern platforms provide access to different employees involved in the
project.
The market for data science platforms is growing along with the market for artificial
intelligence and advanced data analytics. According to Markets and Markets, the market
for big data analytics platforms is growing by an average of 30% per year. There are new
products that developers call data analysis platforms or data science platforms, and it
can be difficult for an unprepared person to understand them.
Real estate market. The use of big data allows you to improve the sale of real estate.
For example, using analytics tools designed for realtors helps them analyze their
audience and target buyers.
Entertainment, content. Consider the example of an online cinema. The Big Data analysis
platform helps the user find a movie that will definitely interest him. But you need to
look deeper - Big Data also helps to understand what films to make for moviegoers.
Agricultural industry Sensors, video cameras, sensors and other equipment help to
collect information about crop yields, weather, soil conditions, air humidity, livestock
growth. Big Data analysis helps to optimize the work of farmers.
Advertising and online trading. Big Data analysis systems examine the behavior of
visitors to an online store, compare the information received with the information
already available, and help predict which product a particular person may pay attention
to. As a result, advertising can be used more effectively.
Security. Any online business needs to deal with threats in a way that is as invisible
to the user as possible. The Big Data analysis system and machine learning allow
modeling the "respectable behavior" of website visitors. As a result, you can quickly
detect potentially dangerous, suspicious users and take the necessary measures.
The medicine. Big Data analysis platforms have been working in medicine for a long time
- they collect, structure, allow sharing and provide doctors from different parts of the
world with access to research, diagnostic methods, treatment, and disease prevention.
State administration. The Big Data analysis system helps the country's government make
important decisions in various sectors (health, economy, employment, crime control,
security, emergency response).
Internet of things. Big Data and IoT are closely related to each other. Devices
connected to the Internet of Things collect huge amounts of information, and the Big
Data analysis system allows you to provide the end user of these devices with exactly
what he needs.
Get in touch
